We use different signal processing techniques and approaches. We work with different neural population signals, continouous time signals such as local field potentials (LFP) and EEG, as well as point processes such as multiunit acitivty. Especially in combination these signals can be of informative in a complentary fashion, with different strengths and weaknesses, for example regarding spatial resolution and the identification of their sources.
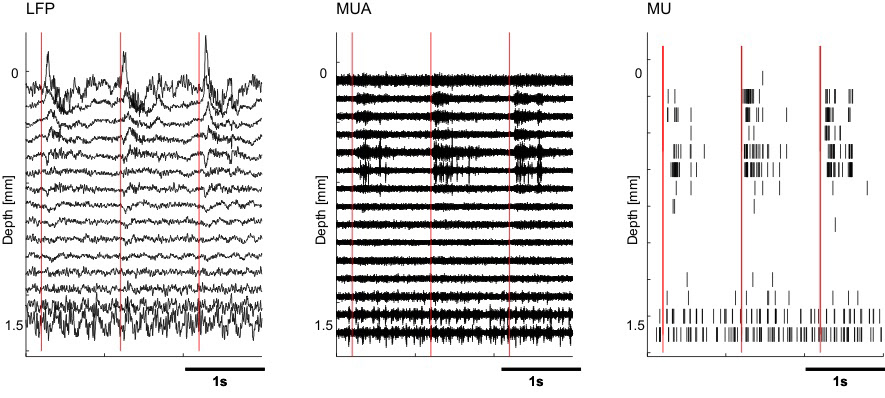
In general, the recording of neural activity with multielectrode arrays produces multidimensional data. And any analysis approach comes down to a problem of the right amount of dimensionality reduction. And depending of the research question, to allow for an adequate description and quantification of the investigated variable. Important dimensions are obviously the position and location of the electrode in the respective part of the brain at a specific depth, and of course the time course of the signal. Necessary of course is also an population estimate, by measuring several subjects on order to assess the variability of the experimental variable under consideration.
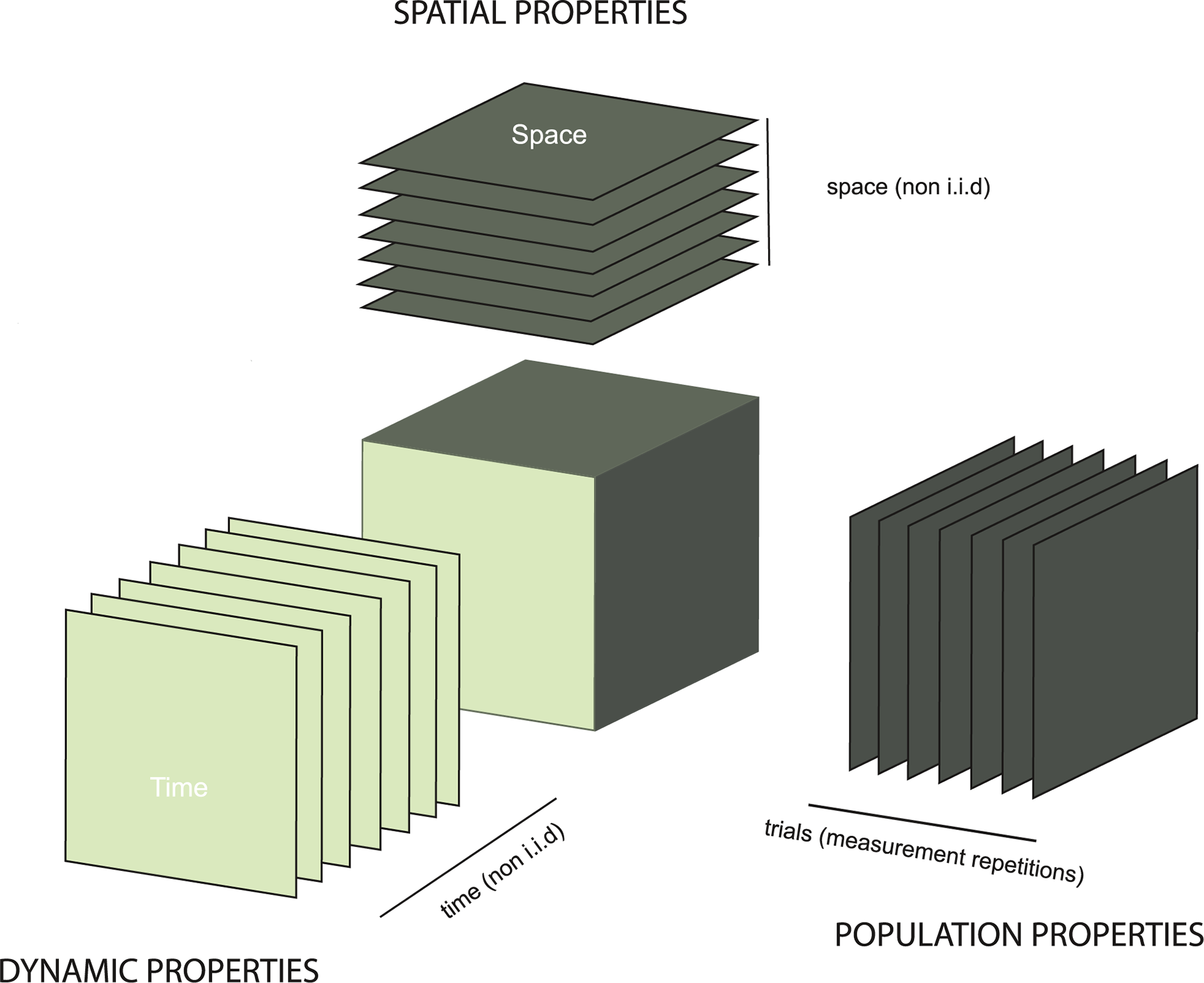
Sketch of data analysis, illustrating different dimensions of the electrophysiological data sets.
Some principles...
We follow a 'philosophy' in terms of data analysis, with some core principles, that we think to be valuable, most of the well known anyways. Of course, there are always exception to the rule, and these principles are also not strict rules anyways.
1) First and foremost: shit in - shit out. That means the most important thing is to acquire and measure good data. Good data usually requires a lot of time, patience, and repetitons, as well as a lot of thought. It is important to keep this in mind. Quick-and-dirty approaches might sometimes be adequate, but in most cases don't believe anybody who says they are equal. So this means it is important to produce good measurements and high quality data. This may take a lot of time. However, with good experiments and data, data analysis becomes quite easy. And there is no single signal processing technique of transfomration can recover missing or shitty data.
2) Small sample sizes are not reliable (obviously). There are clearly cases, where there has to be made a choice between small sample size or no sample size at all. But it is necessary to be able to repeat your experiment, whenever you are asked to. It should not be difficult for you to simply replicate the data (besides the additional time effort). But if you can't do that, there is something wrong with your approach, and justifying it with small sample numbers won't help.
3) There is no way around in doing the experiments yourself. There is a lot of hidden knowledge and discoveries hidden in actually performing the experiments in all its practical aspects. So you learn and generate new ideas while performing the experiments and learn from experience. And not everything has to be documented. If you sit hundreds of hours watching brain activity, you have implicit knowlege which can guide you. That is trivial, but is sometimes forgotten, when everything has to be noted down somewhere. Trust your instincts.
4) Per se data analysis is mostly a transformation (or rearrangement) of numbers. So everything is already in the data (see 1)). The data is simply re-ordered by some fixed rules, hopefully to shape out some information. However, if it was not in the data before, it will not be in there later. No matter what transformation will be done. Deal with it. There a many reasons why it is not, but if you can't show the results simple and straightforward, then maybe it is necessary to redesign the experiment. There is no way to get a result, by going on a analysis spree with such sophisticated approaches, that it basically invented your own new language, that noboday except you can udnerstand anymore.
5) There will be always somebody saying that these experiments are easy. But it is essentially difficult. And it is essentially a slow process. There is also rarely a quick detour. But there is no way around in at putting in the work And usually when you think that it is good enough, there is surely many ways to improve it. But never forget, usually you try things where you and nobody knows what is supposed to happen, so be observant. The most dangerous situation is when people try to force you into a quick and dirty approach, and complain why evreything takes so long, if they themselves do not do the work. Either get in the lab and work on it. Or let the people do that are in the lab. Its usually the cook who cooks the food, and not the restaurant manager.
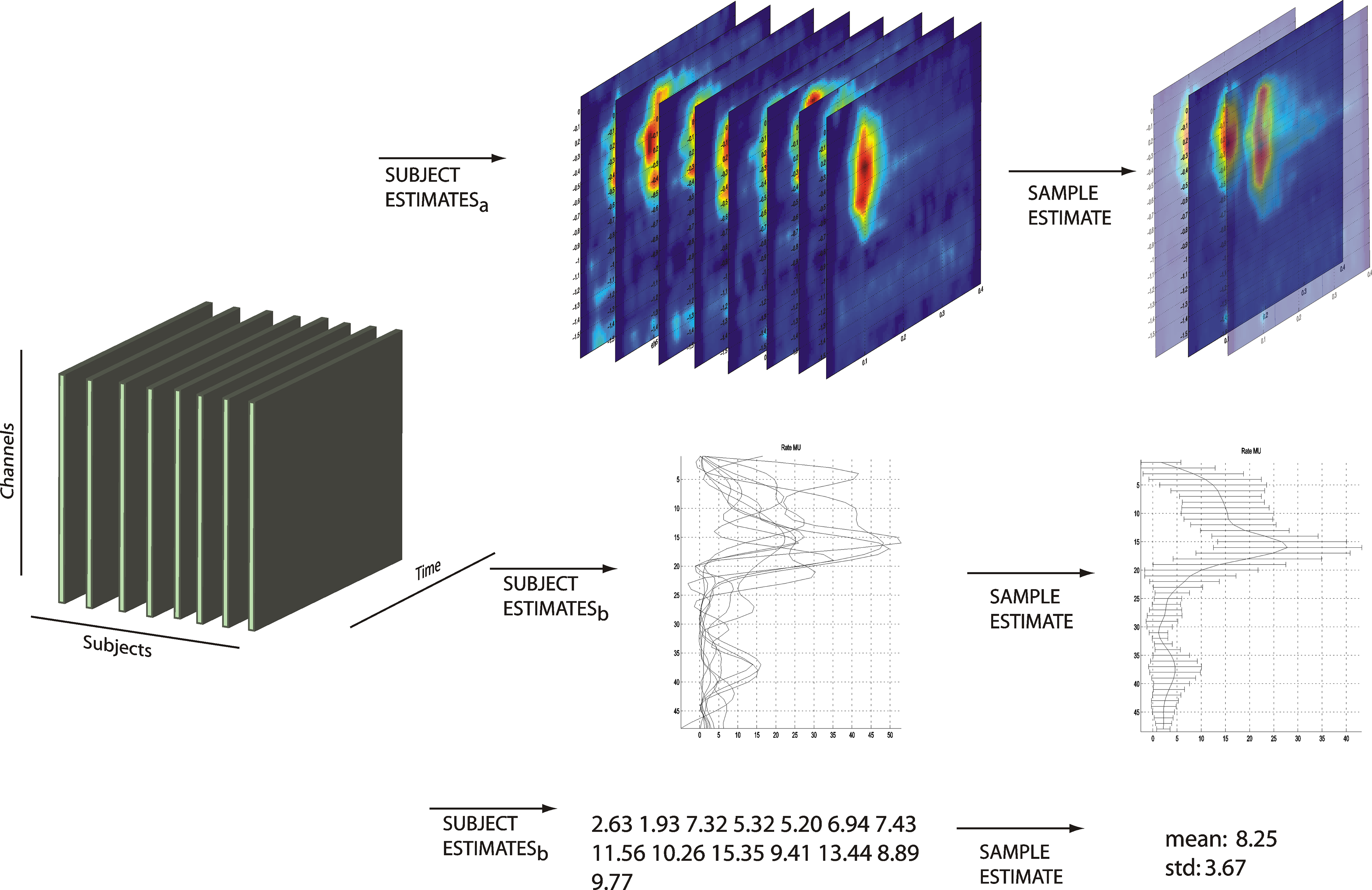
These are only some ideas an exercises and do not necessarily follow strict mathematical rigor, but where more a approach to play with ideas.
Further reading:
Amrhein, V., Greenland, S. & McShane, B. Scientists rise up against statistical significance. Nature 567, 305–307 (2019).
Ioannidis, J. P. A. Why Most Published Research Findings Are False. PLoS Med. 2, e124 (2005).